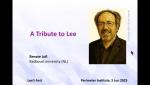
Search results
49 - 60 of 16890 Results
Format results
-
-
Battle of the Big Bang: The New Tales of Our Cosmic Origins
Niayesh Afshordi University of Waterloo & Perimeter Institute
PIRSA:25060061 -
Q&A/Panel Discussion: Time in Loop Quantum Gravity
-
Laurent Freidel Perimeter Institute for Theoretical Physics
-
Simone Speziale Aix-Marseille University
-
Etera Livine French National Centre for Scientific Research (CNRS)
PIRSA:25060051 -
-
Bulk-Boundary in Spinfoams
Etera Livine French National Centre for Scientific Research (CNRS)
PIRSA:25060048 -
Quasi-topological principle and its degrees of freedom
Simone Speziale Aix-Marseille University
PIRSA:25060049 -
-
Q&A/Panel Discussion: Cosmology, Phenomenology and Time
PIRSA:25060076 -
Tempus Discretum: On the nature of cosmic time
Niayesh Afshordi University of Waterloo & Perimeter Institute
PIRSA:25060046 -
Dark Matter as the leftover of historical violations of the Hamiltonian constraint
Joao Magueijo Imperial College London
PIRSA:25060045 -
GRB neutrinos and quantum-gravity-induced in-vacuo dispersion
Giovanni Amelino-Camelia University of Naples Federico II
PIRSA:25060044 -
Lee Smolin: His Important Critical Role with Regard to Unconventional Physical Theories
Roger Penrose University of Oxford
PIRSA:25060075