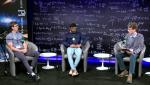
193 - 204 of 16890 Results
Format results
-
-
LitLLMs, LLMs for Literature Review: Are We There Yet?
Gaurav Sahu Mila - Quebec Artificial Intelligence Institute
PIRSA:25040076 -
Gold-medalist Performance in Solving Olympiad Geometry with AlphaGeometry2
Yuri Chervonyi Deep Mind
PIRSA:25040075 -
Human Level AI by 2030
Jared Kaplan Johns Hopkins University - Department of Physics & Astronomy
PIRSA:25040074 -
-
-
Lecture - Cosmology, PHYS 621
Neal Dalal Perimeter Institute for Theoretical Physics
-
-
Beyond Articles: Three Pillars of Scientific Transformation
Oleg Ruchayskiy Niels Bohr Institute
PIRSA:25040067 -
-
Accelerating Discovery: Mapping the Future of AI-Enhanced Theoretical Physics
Axton Pitt Litmaps
PIRSA:25040065 -
The Non-linear Early Universe
Dani Figueroa Instituto de Fisica Corpuscular (IFIC)