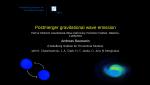
Post-Merger Gravitational Wave Emission
Andreas Bauswein Max Planck Institute for Astrophysics (MPA), Garching
Andreas Bauswein Max Planck Institute for Astrophysics (MPA), Garching
William East Perimeter Institute for Theoretical Physics
Masatoshi Yamada Universität Heidelberg
Zhi-Wei Wang University of Waterloo
Robert Mann University of Waterloo
Anders Eller Thomsen CP3-Origins
Astrid Eichhorn Universität Heidelberg