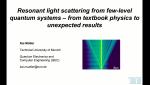
Displaying 73 - 84 of 4948
Format results
-
-
-
-
Quantum simulation for materials modeling
Alexandre Cooper-Roy Institute for Quantum Computing, University of Waterlo
-
Quantum simulation and computing with Rydberg atoms
Johannes Zeiher Max-Planck-Institut für Quantenoptik
-
Towards quantum simulation of vacuum decay
Matthew Johnson York University
-
Efficient quantum simulations with quDits
Christine Muschik Institute for Quantum Computing (IQC)
-
-
Measurement-induced phenomena and protocols
Timothy Hsieh Perimeter Institute for Theoretical Physics
-
-
-